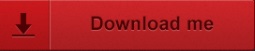
In all experiments, we compute mel-spectrograms with 128 mel bins, an FFT size of 1024, and a 256 sample hop length. 4.2 Model Architectures and Hyperparameters Table 1: Mean Opinion Scores in a human listening test. When evaluating, we apply the same treatment (low-cut filter at 35 Hz and normalization) before applying our enhancement models.
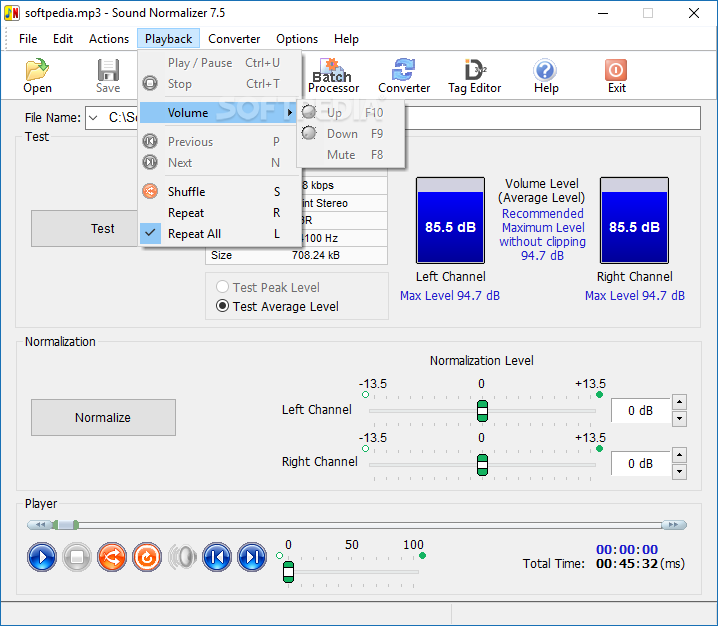
We find that this treatment helps improve our models’ training stability. As a final step, we apply a low-cut filter to remove nearly inaudible low frequencies below 35 Hz and normalize the waveforms to have a maximum absolute value of 0.95. For simulation of low-quality recordings, we source room impulse responses from the DNS Challenge dataset and realistic background noise from the ACE Challenge dataset. Using the procedure described in Section 3.2, we generate a dataset of high- and low-quality recording pairs. This sample rate has shown to be favored by most speech enhancement work and can be potentially super-resolved to 48 kHz with bandwidth extension techniques. We start by downsampling our data to 16 kHz following the setup of prior vocoding work.
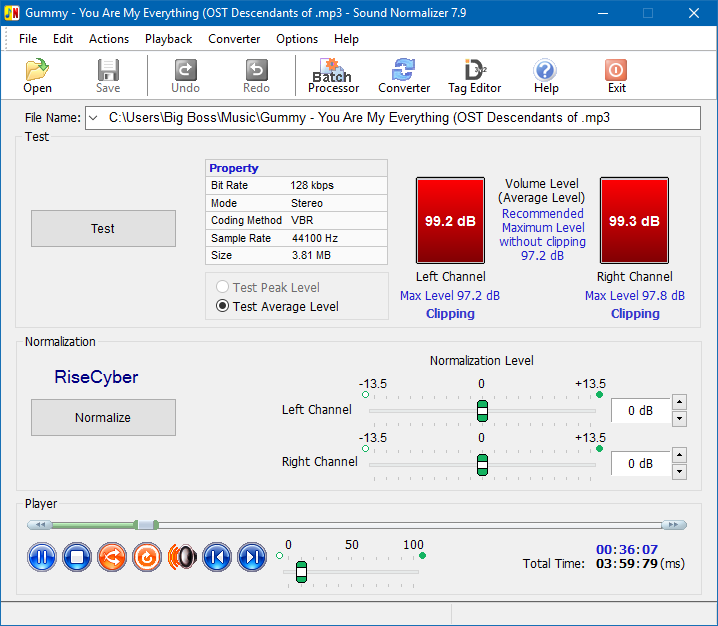
We use 5841 samples for training, 3494 for validation and the rest for testing. We exclude the distorted electric guitar samples to avoid fitting our models to production effects.
Sound normalizer 2.98 professional#
We train and evaluate models on the Medley-solos-DB dataset, containing 21,572 three-second, single-instrument samples recorded in professional studios. Therefore, we aim to develop a solution that works for polyphonic signal enhancement and reflects the unique qualities of music perception. Moreover, the perception of music quality typically differs from that of speech.įor example, human listeners may find reverb pleasant in music, while it is usually undesired in speech.
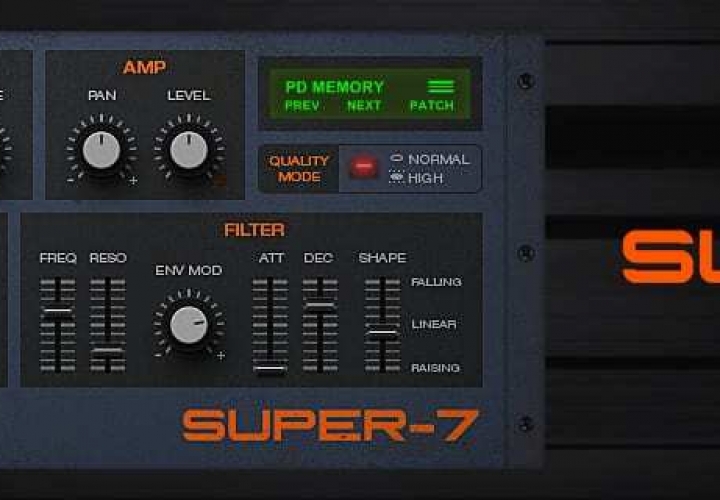
However, music signals are often polyphonic, i.e., there can be an arbitrary number of sources to be extracted at once. In speech enhancement, end-to-end methods such as HiFi-GAN and Demucs achieve this by extracting the speech source from a mixture of sources. A solution that faithfully transforms a low-quality recording into what it would sound like recorded professionally must implicitly or explicitly infer all of these aspects from the signal alone. Additionally, acoustic properties such as the size, shape, and reflectivity of the recording environment vary between different recording setups.įinally, background noise is hard to capture and generalize, especially non-stationary noise. Parameters of the recording device, such as frequency response characteristics, vary drastically across different hardware. The main difficulty of such an endeavor is that so many aspects of the low-quality recording setup are unknown.
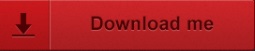